How PepsiCo is using predictive analytics to streamline product development /
PepsiCo is working with tech startup Black Swan to use machine learning to predict and respond to emerging trends.
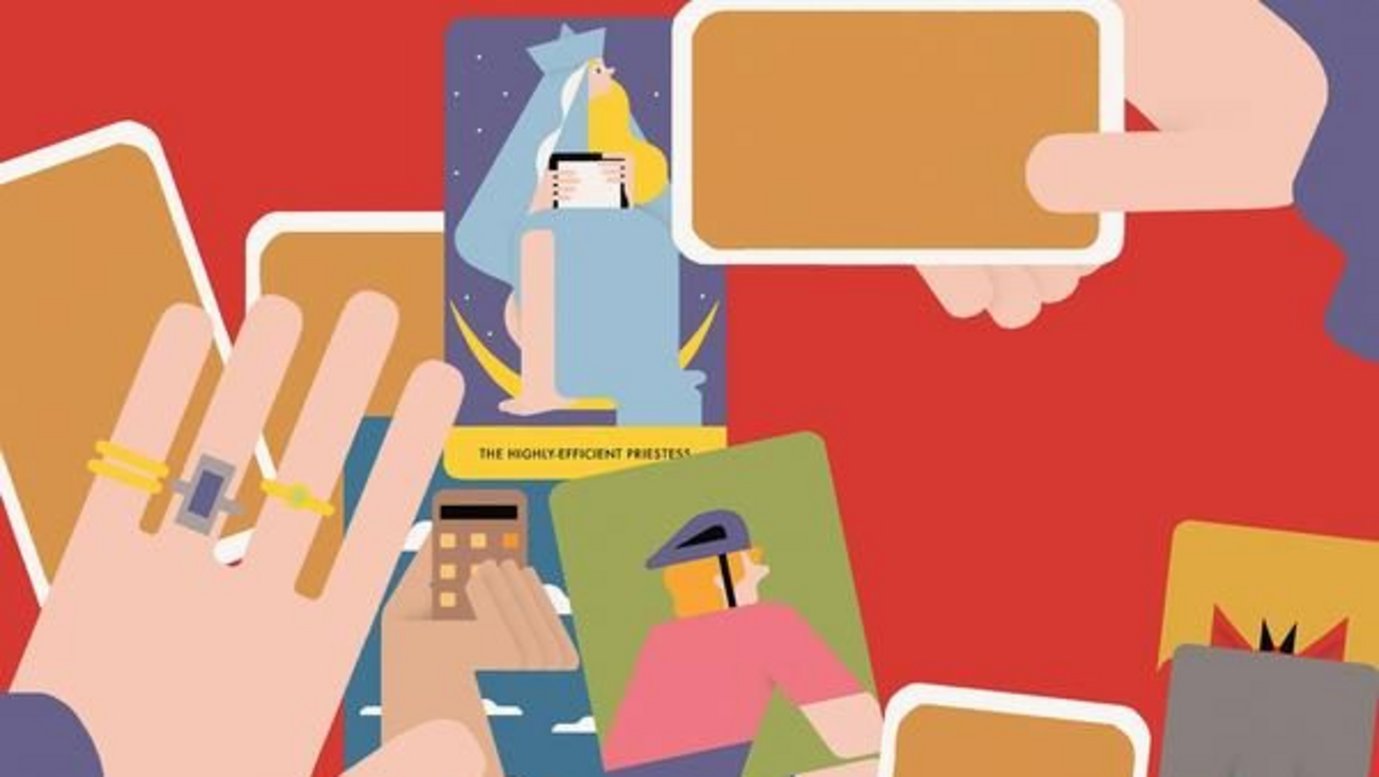
At an open house session during MRS Impact Week, James Howarth, strategic insights director at PepsiCo, explained how they’d used Black Swan’s predictive analytics to develop new flavours of Sensations crisps. Black Swan’s Trendscope uses social and historical data to identify what ingredients are currently trending, and predict which ones will scale in the next six to 12 months.
PepsiCo used the Trendscope to help pick out the new Sensations flavours. They have yet to launch, but when they were tested with a group of consumers, the test scores were much higher than usual. So high that PepsiCo didn’t have to go back to the drawing board, alter the recipes to cater to the feedback and re-test them, as it usually has to. Instead, it could move onto the next stage, cutting the development time in half.
So, while PepsiCo doesn’t yet know whether the crisps will sell better than others, the predictive analytics has already saved the brand time and money.
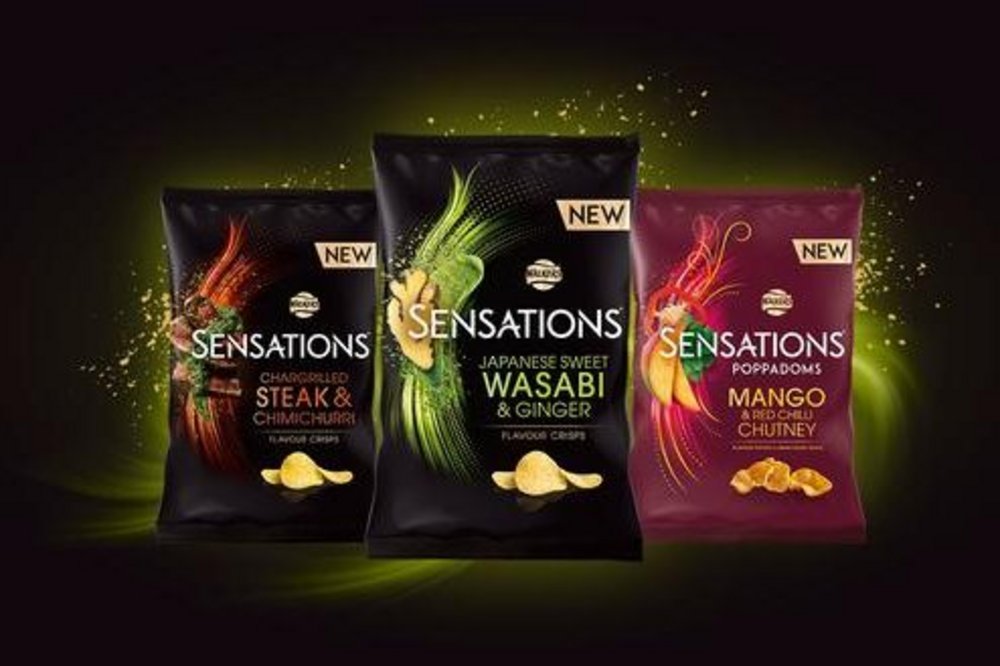
We spoke to Howarth and Black Swan Data co-founder Hugo Amos, to find out how the companies are working together and what impact it’s had on both internal culture and business success.
Why did you think it was important to use data and predictive analytics at PepsiCo?
Howarth: We know that consumer demand is fragmenting, so what people want to buy is becoming more specific, more niche, more individualised and we need to respond to that because that’s where the growth opportunities lie. It’s constantly evolving and what we’re seeing is 10/20/30/40 competitors, products, ingredients emerging at any one point, but only a couple of those end up scaling.
Now the important thing for us is not just to say to our sales and our marketing teams: ‘here are all the things that are trending’ because it just drives chaos within the business. What you need to be able to say to them is ‘here are 20/30/40 things that are all trending, but here are the 2/3/4 that we want to place our bets behind’.
It allows you to do two things. First, you get ahead of your competitors, because you’re being predictive so you’re going to be activating against those trends a lot sooner than some of your key competitors. And second, it reduces noise. It adds simplicity to your business by saying here are the couple of things that we have data and evidence and consumer opinion behind and why you should act on them.
Where do you get all the data from?
Amos: Anywhere and everywhere to be honest. We have partnerships with lots of data providers and owners of data. Social data is useful to us, so we have direct relationships with companies such as Twitter and other providers of that kind of data.
Depending on the things we are predicting, we use different datasets. So we work a lot with different datasets like contextual data, weather and events data and points of interest data. We also do loads of interesting work with maps and footfall and tracking people’s movement on mobile devices. We just use whatever data we need to better understand the consumer.
How do you process the data? Do you identify trending ingredients and then find ways to use them in new products? Or do you look at the reasons why certain ingredients are trending and then develop products based on those insights?
Howarth: That’s where it all starts to piece together. We say: ‘What is really starting to trend? What do we see scaling over the next 12 to 18 months? And what do we do about that?’
Then for some things we might have a right to succeed, so we have an opportunity to incubate a smaller brand created based on what’s trending. And we’ll put it into the right kind of channels to grow. That’s one response, the other one is to say: ‘we’re seeing a bunch of these trends that all ladder up to say something, like sustainability, is really key’. So we start to put all those together and then discuss how we can talk about that trend motivator with our bigger brands.
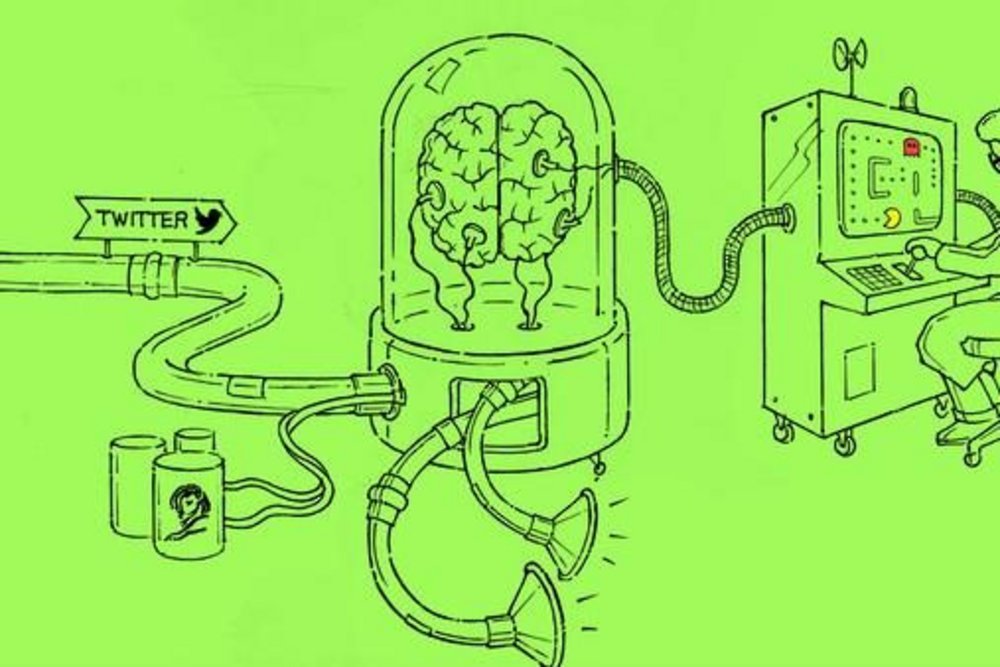
Does PepsiCo have a specific dataset in the Black Swan system?
Amos: PepsiCo has certain datasets that power the intelligence for them, other brands would have slightly different datasets. Each time we work with a new client or category the dataset is everything, so some of it will be data we already have and the rest will be historical data that we’ll buy to supplement it.
The thing to understand about data is that each category is slightly different. So, for a consumer goods brand like PepsiCo or Unilever, data about what people talk about on the internet is a really powerful source of information. If you’re a pharmaceutical company looking at drug innovation, however, it’s not going to be as relevant – they’re going to be looking at more synthesising, proprietary research datasets. The same tools can be applied to different datasets but it depends on what problem you’re trying to solve.
How do you know your predictions are accurate?
Amos: The algorithm is trained on seven years of data and within that we get to over 90% accuracy on a six-month projection, and then you get a degradation of that accuracy as you look further into the future. But the way that we actually understand whether we are doing the right thing is by using historical data to go back and look at what trends happened in the past – so we run the algorithm on things we know have happened and see if it accurately predicts them. The other way we know whether the predictions are accurate or not is whether we see the trends actually happen.
Everything we do at Black Swan is about making the length of that prediction longer, that’s where we’re focusing. Now the tool is configured to a very accurate six-month prediction and we can go longer than that, but the way you go longer is by having better data – more training sets of data means more accuracy.
What’s the difference between ‘big data’ and ‘thick data’?
Howarth: Big data is what these guys are experts in: big, digital datasets. But we still believe there’s a massive reason to have traditional data gathered from individual consumers. So, when we say ‘thick data’ we’re talking about one or two hundred consumers that we have a relationship with that we interact with via research groups or through digital platforms.
We ask them questions to get a real depth of understanding around individual trends – if some people on the panel are eating insect protein, for example, and we’ve seen that as a trend, we’ll ask them why they are doing that, what it is that’s pushing them to eat insect protein. And that information is what allows us to create a framework around what these trends really mean. So, we think about it like so: the big data tells us what’s going on and the thick data helps us understand the why.
So the big data tells you which questions to ask?
Howarth: It can do a number of things, it can tell you which questions to ask, but it can also be very prescriptive about what is really going to take off in the next six to 12 months. Does PepsiCo have a specific dataset in the Black Swan system?
Amos: PepsiCo has certain datasets that power the intelligence for them, other brands would have slightly different datasets. Each time we work with a new client or category the dataset is everything, so some of it will be data we already have and the rest will be historical data that we’ll buy to supplement it.
The thing to understand about data is that each category is slightly different. So, for a consumer goods brand like PepsiCo or Unilever, data about what people talk about on the internet is a really powerful source of information. If you’re a pharmaceutical company looking at drug innovation, however, it’s not going to be as relevant – they’re going to be looking at more synthesising, proprietary research datasets. The same tools can be applied to different datasets but it depends on what problem you’re trying to solve.
How do you know your predictions are accurate?
Amos: The algorithm is trained on seven years of data and within that we get to over 90% accuracy on a six-month projection, and then you get a degradation of that accuracy as you look further into the future. But the way that we actually understand whether we are doing the right thing is by using historical data to go back and look at what trends happened in the past – so we run the algorithm on things we know have happened and see if it accurately predicts them. The other way we know whether the predictions are accurate or not is whether we see the trends actually happen.
Everything we do at Black Swan is about making the length of that prediction longer, that’s where we’re focusing. Now the tool is configured to a very accurate six-month prediction and we can go longer than that, but the way you go longer is by having better data – more training sets of data means more accuracy.
What’s the difference between ‘big data’ and ‘thick data’?
Howarth: Big data is what these guys are experts in: big, digital datasets. But we still believe there’s a massive reason to have traditional data gathered from individual consumers. So, when we say ‘thick data’ we’re talking about one or two hundred consumers that we have a relationship with that we interact with via research groups or through digital platforms.
We ask them questions to get a real depth of understanding around individual trends – if some people on the panel are eating insect protein, for example, and we’ve seen that as a trend, we’ll ask them why they are doing that, what it is that’s pushing them to eat insect protein. And that information is what allows us to create a framework around what these trends really mean. So, we think about it like so: the big data tells us what’s going on and the thick data helps us understand the why.
So the big data tells you which questions to ask?
Howarth: It can do a number of things, it can tell you which questions to ask, but it can also be very prescriptive about what is really going to take off in the next six to 12 months.
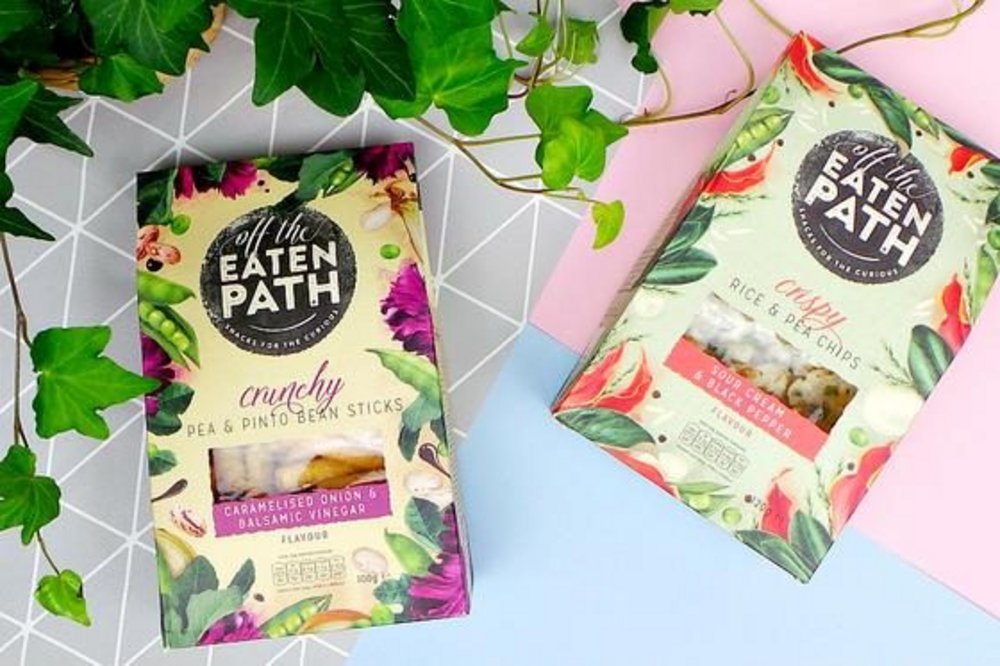
How close are you to bringing a product to market based on this predictive data?
Amos: There have already been PepsiCo products created based on the data. One that was in the public domain was made in partnership with Unilever and the Lipton Ice Tea brand. They launched a Matcha Ice Tea last year, which was based on this methodology and there are several others in the pipeline.
Howarth: There is another one in the UK called Off the Eaten Path, it’s a smaller brand that we launched in partnership with Sainsbury’s and we’ve seen amazing sales results. Sainsbury’s wants to scale it up across more stores and the other retailers want to get it on their shelves as well.
The Trendscope helped us identify flavours and some of the ingredients that we put in the Off the Eaten Path products. It’s quite cool because it’s a small brand so we can do much more agile innovation development, so from concept to shelf took half the time of what it would normally.
If you’re picking a new flavour, does it ever produce anything surprising?
Howarth: It depends. If you’re thinking of everything through a certain lens, like if you’re a milk producer and someone told you to go make something with insect protein, you’d be pretty surprised. But with a business like PepsiCo, which sells juice, oats, snacks, water, CSDs [carbonated soft drinks], etc., there’s nothing that could make you think ‘that’s crazy, we could never utilise that’ because somewhere across our brands there’s probably a rationale for it.
If you walked around Shoreditch and asked people, you’d probably hear about a lot of them anyway – there’s nothing that you won’t have heard of before. And if there was, we’d be worried because if you told me something was going to scale in six months and I’d never heard of it, it’s not the right thing. It’s not about spotting millions of things early on that no one has heard about, it’s about figuring out which ones are going to grow.
It must help to have data when you’re trying to convince someone to back something?
Howarth: That’s where this is completely transformative culturally, there’s a classic quote: ‘Without data you’re just another person with an opinion’. And historically if you think about front-end innovation, it’s just people sitting in a room coming up with opinions, and then you go to your senior leadership and they ask you why – and you’d end up explaining that it’s just something that you thought.
This gives a massive justification for why you’d do something, we’re saying: ‘this is trending with our consumers and it’s going to be big over the next six months or so’. That’s why we’re not surprised when we get great consumer test scores because we already knew it was popular, and it gives us great justification as we go through our innovation life cycle.
Want more of the same? /
We don’t just write about best-in-class campaigns, interviews and trends. Our Members also receive access to briefings, online training, webinars, live events and much more.